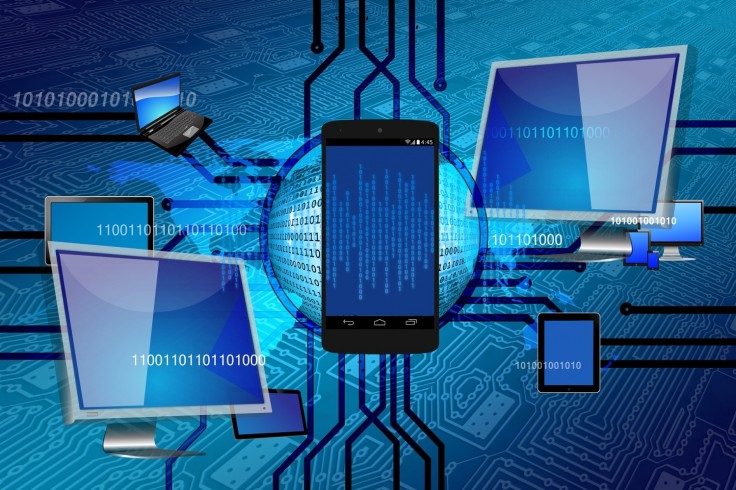
Over the course of recent years, as an increasing number of organizations and enterprises welcome the inclusion of artificial intelligence and machine learning- it appears as though companies have only just begun to realize the potential that these 'modern' technologies have.
Not only can AI and ML positively influence the daily operations of a company, but it can also leverage the best aspects of the technology to aid employees in making efficient decisions within a shorter span of time.
Having said that, however, as more and more enterprises reap the benefits offered by ML and AI, it is equally important that we realize that the advantages offered by these technologies are only as good as the foundations they're based on, or more specifically- good data quality.
If an organization were to rely on 'bad data' to be fed into their AI and ML algorithms, they could potentially bear witness to their companies toppling down, since bad data creates massive hindrances in the proper functioning of AI and ML. Case in point: a recent report by Web Hosting Data UK found that more than two thirds - roughly 68% - of web hosting providers based in the UK had made "bad data" decisions in 2019. Furthermore, increased reliance on poor quality data also plays a key part in the business slagging inefficiency, and consequently, losing its reputation.
Luckily, however, there's a new area for exploration- namely, the automation of data quality, so that it lies in tandem with AI and ML requirements. Additionally, an increasing number of professionals are also working on the potential that AI and ML have in the automation of data quality.
In an attempt to aid our readers better grasp the potential that artificial intelligence and machine learning has in automating data, we've compiled an article that provides a comprehensive answer to the question - can data quality be automated by AI and ML?
Before we get into answering the question, however, we'd like to bring our readers to terms with the plenty of risks associated with poor data quality.
What are the Risks of Relying on Poor Data Quality?
For the sake of reaping as many benefits from the datasets at an organization's disposal, it is essential that they realize the perils of poor data quality. Typically, within the context of the present-day IT landscape, companies tend to rely on ML in a multitude of situations, including everything from movie-streaming services, several top options trading platforms, to helping supermarkets arrange and coordinate their shelves.
With that being said, however, the deployment of the machine learning algorithm could wreak a lot of damage, particularly if the algorithm is set to work amidst the foundation of poor data quality.
To further demonstrate the far-reaching consequences of increased reliance on poor data quality, let's consider an example. If organizations were to rely on ML-centric algorithms to work on the discovery and testing of pharmaceuticals, one can only assume the dire impact that a single error in the chemical compound data would have on the stimulated drug testing. Not only does the organization risk closing down, but drug testing could also result in the loss of life.
Similarly, the problem presented by a poor quality dataset is also made evident with self-driving cars. As an emerging application, the entire existence of a self-driving car, particularly how well it bodes in the future, depends primarily on the data set from which the car derives its maps, addresses, along with responses to the other vehicles on the road. Needless to say, a poor quality data set could result in, at best, loss of brand reputation, and at worst, multiple road accidents and loss of life.
When it comes to formulating a set of precise rules and calculations, otherwise known as machine learning algorithms, organizations need to realize they've basically got two options available. The first and much more preferred option is that companies deploy ML algorithms to automate the improvement of data quality, or to come to terms with the possibility that poor data quality could throw off their entire plans for the future.
How Can Organizations Automate Data Quality?
On paper, the digital transformation from manual to automated, and then "intelligent" data quality appears to be much simpler than the process actually is. For starters, one of the most fundamental notions that enterprise owners need to come to terms with is that reaching the "intelligent" level of data quality management requires a long-term plan and a consistent effort.
Moreover, organizations should have a clear-cut definition of what they hope to achieve by "intelligently automating'' their data so that they know what to expect. Many SaaS companies now rely on some form of automation as a core feature of their business model, but what does that mean, exactly? Simply put, intelligently automated data refers to a reliance on systems and processes to help the individuals responsible for data quality realize what their greatest concerns are.
In addition to regularly reviewing key performance metrics to recognize certain trends in data quality, organizations need to dig deeper to truly gain a correct understanding of their data quality. To gain a true understanding of an organization's data quality, we'd suggest looking at overall completion rates of key attributes, along with surveilling for any timing issues, particularly in the data receipt and data load stages.
Once organizations are done with the monitoring phase, they can move on to the next steps, which contain the much-awaited elements of automation, testing, and learning. Usually, once the surveilling phase is done with a data quality program, the next phase usually deals with the evolution of machine learning to automatically recognize and respond to a multitude of data types.
Simply put, the ultimate goal in a data quality program is for the ML algorithm to automatically improve the data quality over time. Furthermore, while an organization seeks to fulfill this goal, they learn to prioritize complex details over simple ones. One such example is in the instance that companies group commercial entities together, a successful data automation program can look for simple details such as the company's name, along with the more complex details such as head office addresses, CEO names, etc.
Concluding Words
At the end of the article, we can only hope that we've cleared our reader's mind over some of the confusion that they might be having data quality. Taking into account the adverse influence that poor data quality has on an organization, we believe it crucial to an organization's proper functioning that immediately starts on improving their data quality!
Author's Bio:
Shigraf is a tech writer and editor at PrivacyCrypts, who has a passion for technology. She pours her passion for writing on topics regarding cybersecurity and AI. Follow her on twitter.
Gravatar Email: shigrafaijaz@gmail.com