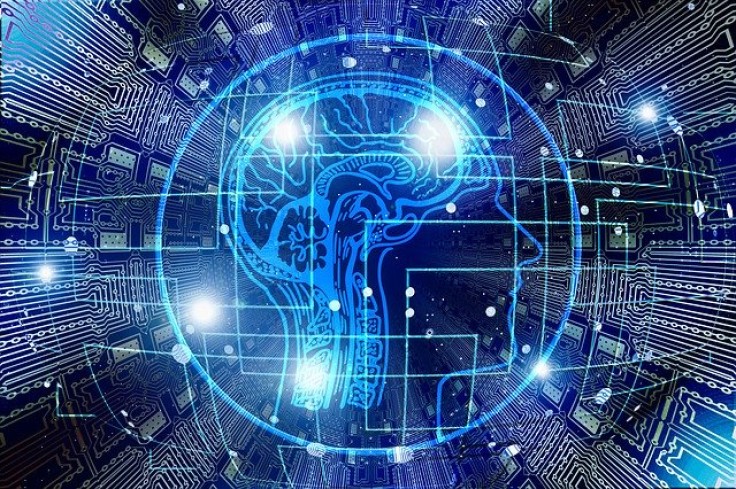
A machine learning system developed by MIT researchers can either predict a specific task or defer the decision making to a human expert.
Artificial intelligence and machine learning have been widely used by a variety of industries, including healthcare. In the medical field, researchers have utilized machine learning to help experts better diagnose cancer.
The difficult part about this hybrid approach is knowing when to rely on a human expert and when to let the machine decide. This issue is not merely concerned about who performs the task better. When human experts have limited bandwidth, the machine may have to be trained to minimize the frequency of having to ask for help.
Read also: Solar Energy Trading Platform Being Developed by Singapore Start-Up Company
Researchers from the MIT's Computer Science and Artificial Intelligence Lab (CSAIL) developed a machine learning system to address this issue. The system can adapt the timing and frequency of deferring to human experts. The decision will be based on factors including level of experience and teammate's availability.
CSAIL researchers trained the system on multiple tasks. The tasks include looking at chest X-rays. They also trained it to diagnose conditions such as lung collapse and an enlarged heart. In the case of an enlarged heart, the human-AI hybrid model performed eight percent better than either could perform on their own.
Ph.D. student Hussein Mozannar is the lead author of the study. He is joined by David Sontag, who is the Von Helmholtz Associate Professor of Medical Engineering.
Mozannar said that in medical environments where doctors do not have many extra cycles, looking at every single data point from a particular patient's files is not an ideal use of their time. In that scenario, the system needs to be extra sensitive to the expert's time and ask for help only when it is absolutely necessary.
The researchers divided the system's part into two. The first part is a classifier. It can predict a certain subset of tasks. The other is a rejector. The rejector decides whether to let either its own classifier or a human expert handle the task.
David Sontag said that their algorithms allow optimization for whatever choice one wants. That can be the specific prediction accuracy or the cost of the human expert's time and effort. And by interpreting the learned rejector, the machine learning system provides insights as to how the human experts decide and in which settings may artificial intelligence be more appropriate, or vice-versa.
For the research, they had developed a series of artificial experts. This approach enabled them to tweak parameters like experience and availability. The researchers plan to test the system with real human experts such as a radiologist. They will also look into developing systems that can learn from biased expert data. Also, they will explore the possibility of developing systems that can work with multiple experts at once.
Sontag said that they hope that the method they have developed will inspire machine learning practitioners to be more creative in incorporating real-time human expertise into their algorithms.