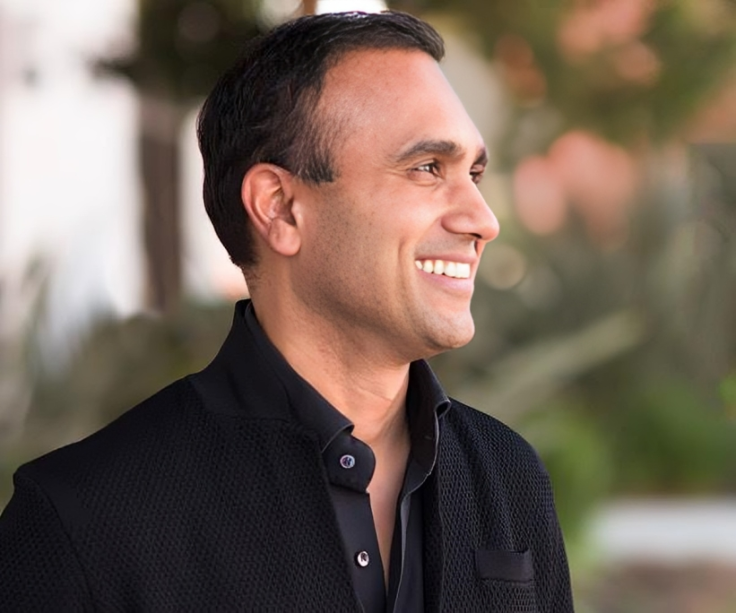
Serial entrepreneur Munjal Shah hopes his newest venture can leverage generative AI to improve patient outcomes in areas such as chronic care and patient navigation.
While the general public has become increasingly familiar with artificial intelligence through chatbot platforms like ChatGPT, some of the more impactful applications of advances in AI may still be flying under the radar. It's fun to ask a chatbot to write a poem or paint like Vincent van Gogh, and it's helpful to draft AI-generated emails, memos, and outlines quickly, but it could be life-changing to use AI to improve health outcomes.
Applying large language models, the neural networks behind the recent boom in generative AI, to health care is the mission of Hippocratic AI. The company is a startup launched by serial entrepreneur Munjal Shah, and it's building an LLM to assist patients with nondiagnostic needs, such as chronic care reminders and direction, dietitian services, and finding and booking appointments with the right specialists.
Hippocratic AI's goal is to leverage the communicative prowess and vast knowledge repository of LLMs. These systems, while not perfect, may offer a significant advantage in their ability to compile and synthesize massive amounts of medical knowledge and use it to generate accurate, patient-specific communication.
"The big idea here is: Wait a minute, we probably only have a few hundred thousand chronic care nurses in the whole U.S. out of the 3 million total nurses, but we have 68 million people with two or more chronic diseases," said Shah in a recent interview for the "Faces of Digital Health" podcast. "Why don't we have 68 million nurses and what would happen to health care outcomes if we did?"
Generative AI and Health Care
Shah founded Hippocratic AI after selling companies to Alibaba and Google. His previous ventures focused on utilizing machine learning and computer vision AI for e-commerce applications. These companies relied on classifier AI, systems that are designed to identify specific patterns and assign data to categories based on those patterns.
Classifier AI might be helpful for categorizing products like shoes or handbags and matching them to consumer preferences, but it's not the sort of generative, multipurpose AI enabled by LLMs. Unlike classifier AI, generative AI can use its training data to create original responses to diverse, unique demands and inquiries, and it can do so in a way that mirrors the tone required for a variety of contexts.
Shah recognized that this type of AI was an ideal fit for the sort of patient-provider communication that can fall through the cracks, given the gap between patient needs and healthcare worker availability.
"I call it super staffing," he said. "This isn't about how we get 10% more efficient. This is about how we 10x the number of healthcare workers. How do we use that to finally deliver better access to health care than ever?"
Shah is quick to point out that the vision for the company is not to replace human nurses with AI but rather to augment existing staffing levels to help fight a growing healthcare worker shortage both in the U.S. and globally.
According to a 2023 study of U.S. nurses by the National Council of State Boards of Nursing, roughly 100,000 nurses left the profession in the past two years, largely due to factors such as burnout and stress. Another 610,388 said they intended to leave by 2027. At the same time, an aging population is leading to growing demand for health care. Hippocratic AI is hoping to both reduce the stress on current nurses and make inroads in filling the gap between availability and need.
"What's the ideal staffing level that would have resulted in the best health care outcomes, even if the math never worked before?" said Shah. "If we got to that staffing level, what would happen?"
Nondiagnostic AI Applications
While optimistic about the potential for LLMs to augment nursing services, Munjal Shah is also keenly aware of the limitations of the technology, notably the occasional generation of erroneous information, which, in a medical context, could have serious implications.
In positioning Hippocratic AI's LLM as safety-based, he's focused only on nondiagnostic applications of the technology. Concerns over generative AI "hallucinating" false statements and presenting them as true have led many to be skeptical of using the technology to make high-risk judgments on patient diagnoses and treatment plans. Even if LLMs are right most of the time, they can't afford to be wrong in situations where a patient's health is at stake. Shah agrees.
"In diagnosis, it'll be unsafe," said Shah. "That's why I said, 'Let's not do diagnoses.' Focus on all the other applications in health care."
"When your test result is negative, do you really need a human to call you up just to tell you they found nothing? Is that a good use of your scarce nurses?"
He cited billing and explanations of benefits as other use cases. Health care in the U.S. is integrated with a complex insurance ecosystem, and it can be difficult for billing specialists, let alone patients, to understand what is covered by their insurance and how much they should be billed for certain procedures.
LLMs are adept at making sense of this sort of convoluted data and communicating it in clear language. Anyone who has asked ChatGPT to explain a dense scientific or technical concept in "simple language" can attest. Shah also points out that Hippocratic AI is trained on a healthcare-specific dataset, and this can include large amounts of both peer-reviewed and evidence-based medical research, as well as exhaustive details of nuanced and varied insurance policies that can be difficult for any human being to keep track of.
The key is to train the model on a trove of well-chosen, domain-specific healthcare data, then subject it to feedback from the human providers trained in the healthcare services that Hippocratic AI's LLM is learning to replicate.
"You need it specifically trained for a domain and that might still not be enough," said Shah. "The other part is you need the medical professionals who do that job today to say it's ready to launch."