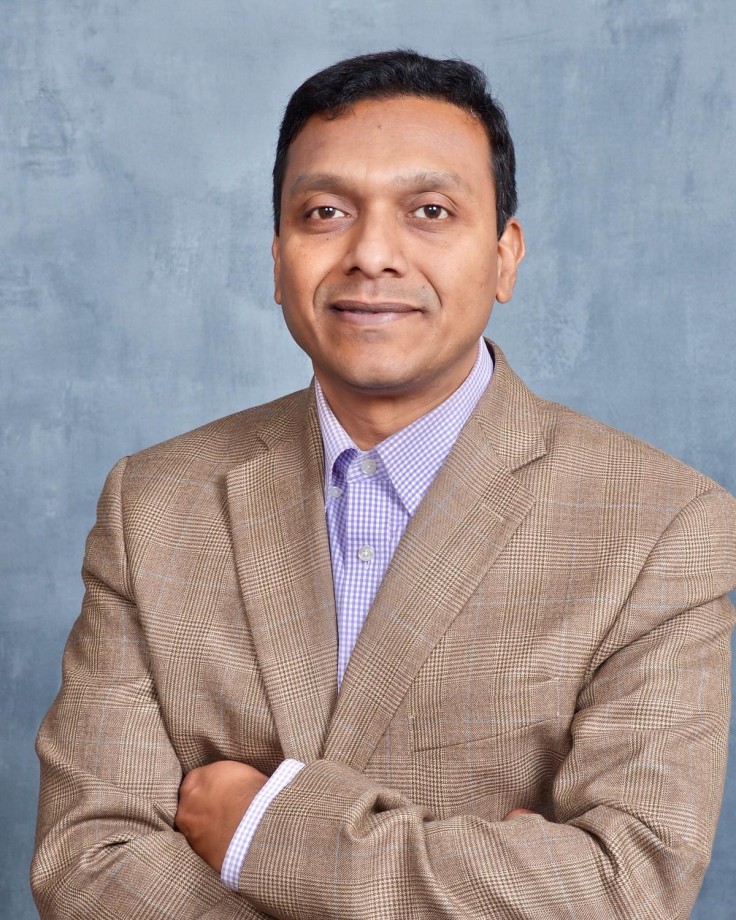
Sanjay Ramdas Bauskar is a Senior Database Administrator with over 23 years of experience in database management, data warehousing, and the integration of machine learning in healthcare. Sanjay's work focuses on leveraging cutting-edge technologies to enhance personalized treatment, early disease detection, and healthcare outcomes. His contributions to healthcare diagnostics are driving the evolution of precision medicine and the application of AI in critical medical contexts.
Abstract: This article explores the significant impact of machine learning algorithms on fetal health monitoring, particularly through the analysis of cardiotocography (CTG) data. The integration of artificial intelligence (AI) into CTG analysis enhances diagnostic precision, facilitating early detection of potential fetal health issues and timely medical interventions. The paper focuses on the challenges faced by high-risk populations, such as patients with breast cancer and diabetes, where traditional monitoring methods may be inadequate. While the use of AI-driven CTG analysis shows great promise, issues such as data variability and the need for generalizability across diverse healthcare settings pose challenges. The article also addresses the ethical considerations surrounding AI implementation in clinical practice. Through the continued advancement of machine learning models, there is potential for improved maternal and fetal health outcomes, as well as broader applications in precision medicine, including breast cancer and diabetes management.
Keywords: Machine Learning, Fetal Health Monitoring, Cardiotocography (CTG), Artificial Intelligence (AI), Breast Cancer, Diabetes Management, Diagnostic Precision, Data Variability, High-Risk Pregnancies, Predictive Models, Healthcare Algorithms, Personalized Medicine, Deep Learning, Medical Interventions, Precision Medicine
The advancement of machine learning algorithms has significantly impacted the healthcare sector, particularly in the field of fetal health monitoring through cardiotocography (CTG) data analysis. This approach leverages sophisticated computational techniques to assess fetal well-being, identify potential health issues, and facilitate timely medical interventions, especially in pregnancies complicated by maternal conditions such as breast cancer and diabetes. Cardiotocography, a prevalent method for recording fetal heart rate and maternal uterine contractions, traditionally relies on manual interpretation. However, integrating artificial intelligence (AI) into this process enhances diagnostic precision, promising improvements in fetal health assessments and outcomes.
Machine learning's application in this domain is notably significant given the challenges faced by patients with breast cancer and diabetes. For instance, breast cancer patients often face limitations in traditional cardiac monitoring methods due to surgery-related complications, whereas diabetes poses additional risks to fetal health. AI-driven analysis of CTG data presents an innovative solution, offering alternative and potentially more accessible means of monitoring fetal and maternal health. This technological intersection is pivotal in addressing the unique diagnostic needs and enhancing the quality of care for these populations.
Despite these advancements, challenges remain in the application of machine learning algorithms to CTG data. Issues such as data variability, generalizability across diverse healthcare settings, and ethical considerations continue to hinder widespread implementation. Additionally, while some machine learning models have demonstrated high accuracy, the ability to consistently identify suspicious fetal states is an area requiring further refinement. Ongoing research aims to bridge these gaps by developing more comprehensive and adaptable diagnostic frameworks that integrate CTG with other diagnostic tools, ultimately improving maternal and fetal health outcomes.
The integration of advanced machine learning algorithms into CTG data analysis underscores a significant shift towards precision medicine, enhancing personalized treatment plans and early disease diagnosis. This approach not only promises to transform fetal health monitoring but also extends its benefits to related fields, including breast cancer and diabetes management, highlighting the broader potential of machine learning in revolutionizing healthcare diagnostics and patient care.
Cardiotocography Data
Cardiotocography, commonly referred to as 'CTG' by medical professionals, is a technique used to monitor fetal heart rate and maternal uterine contractions during labor[1]. The primary objective of using CTG is to assess fetal well-being and detect potential distress, thus ensuring timely medical interventions when necessary. The standard practice involves interpreting these patterns visually, but recent advances have seen the introduction of computerized systems for more precise analysis[2].
These computerized interpretations, often leveraging artificial intelligence (AI) and deep learning technologies, are still evolving. Despite the potential improvements they promise for medical diagnostics, the literature on this specific application of AI remains limited[2]. This scarcity of data highlights the need for further research, especially considering the rapid advancements in machine learning (ML) algorithms that have shown promise in other areas of healthcare, such as cardiovascular risk assessment and genomic analysis[3].
In different healthcare settings, particularly those with limited resources, basic CTG may be more feasible and affordable compared to other fetal assessment tools like ultrasound[4]. However, the integration of AI into CTG systems could potentially enhance their diagnostic capabilities, making them more applicable across diverse medical environments. This development is crucial as it aligns with the broader goal of precision medicine, which focuses on creating personalized treatment plans and early disease diagnosis[3].
Fetal Health Development
Fetal health development is a critical area of study, especially in pregnancies complicated by medical conditions in the mother, such as diabetes and high blood pressure, or conditions directly affecting fetal health and development[4]. Monitoring fetal health involves assessing fetal heart rate (FHR) and uterine contractions, often through electronic fetal monitoring (EFM), commonly referred to as cardiotocography (CTG)[5]. CTG, developed in the 1960s, serves to assess a fetus's health both before and during labor by simultaneously monitoring these key indicators[5]. The monitoring can be conducted externally using devices like a cardiotocograph machine or handheld fetal dopplers[6].
The utility of CTG lies in its potential to identify changes in the fetal heart rate that may signal underlying conditions, such as infections, anemia, or congenital anomalies[6]. In particular, computerized CTG analysis is being explored for its ability to automate the detection of fetal compromise during labor, which could be particularly beneficial in high-risk pregnancies[7]. While CTG is a valuable tool, there remains no definitive evidence that antenatal CTG improves perinatal outcomes. Therefore, further studies are warranted to explore its use in specific high-risk populations[4].
Advanced machine learning algorithms are now being integrated with cardiotocography data to enhance the detection and understanding of fetal health development. These algorithms can potentially identify patterns and markers that might not be immediately apparent through traditional monitoring methods. This intersection of technology and fetal health monitoring aims to improve diagnostic techniques and outcomes, particularly in complex cases involving maternal conditions like diabetes and thyroid disease, which pose increased risks to the fetus[4][5].
Machine Learning in Healthcare
Machine learning (ML) algorithms have significantly advanced the healthcare sector, providing innovative solutions for disease diagnosis, treatment, and prognosis[8]. These algorithms use mathematical models and data points collected from the human body to generate accurate diagnoses[9]. In particular, ML has shown remarkable success in improving the prediction of cancer recurrence and mortality, cardiovascular risk assessment, and enhancing the diagnostic accuracy of radiological investigations such as computerized tomography (CT) scans and magnetic resonance imaging (MRI)[9].
In recent years, ML technologies have been instrumental in the field of fetal health monitoring through cardiotocography (CTG) data analysis[8]. CTG involves recording the fetal heartbeat and uterine contractions, offering crucial insights into fetal health. The integration of machine learning algorithms into CTG analysis has enabled the classification of fetal health into categories such as normal, needs guarantee, and pathology[8]. These algorithms have achieved high accuracy in predicting the pathological state of the fetus, although challenges remain in accurately identifying the suspicious state[9].
A comprehensive review of ML and deep learning (DL) applications within CTG research highlights the ongoing development and refinement of these technologies[10]. The application of AI-driven analysis tools, using both traditional and nonlinear fetal heart rate (FHR) features, has further improved classification accuracy, distinguishing between healthy and pathological fetuses[5]. Despite these advancements, the literature on computerized CTG interpretation based on AI is still evolving, with many systems yet to become commercially available[2].
The implementation of ML in healthcare exemplifies the potential to decrease maternal and fetal mortality rates by automating and enhancing the accuracy of diagnostic processes[11]. These technologies bridge the gap between existing diagnostic methods and the growing need for automatic analysis, paving the way for more integrated and reliable healthcare solutions[5].
Algorithm Development
The development of advanced machine learning (ML) algorithms has been instrumental in improving diagnostic capabilities within healthcare, particularly in the analysis of cardiotocography (CTG) data to assess fetal health. These algorithms utilize a variety of mathematical models and data points from the human body to generate accurate diagnoses and predict health outcomes such as cancer recurrence and cardiovascular risks[9].
In the context of fetal health, ML algorithms are employed to analyze CTG data, which involves assessing fetal heart rate (FHR) features using both traditional and nonlinear methods. This approach enhances classification accuracy and offers more reliable diagnostic information by effectively distinguishing between healthy and pathological fetal states[5]. The deployment of ML algorithms in this domain not only addresses inconsistencies in CTG interpretation but also fills significant clinical gaps by automating the analysis process[8].
A comparative study of different machine learning methods using open-source software such as WEKA has demonstrated the effectiveness of these techniques in terms of accuracy, specificity, sensitivity, and other performance metrics[10]. This analysis is critical as it provides insights into the strengths and limitations of various ML models, helping refine them for better accuracy in diagnosing fetal conditions. Furthermore, algorithms like Random Forests have achieved notable accuracy rates, often outperforming traditional models, thereby underscoring the potential of ensemble approaches in this specific healthcare context[11].
Application in Breast Cancer
The integration of advanced machine learning algorithms in the analysis of cardiotocography (CTG) data presents promising avenues for addressing specific healthcare challenges, such as those faced by breast cancer patients. The prevalence of cancer-therapy-related cardiovascular toxicity (CVR-CVT) has necessitated innovative approaches in cardio-oncology, especially given the limitations breast cancer patients face with conventional diagnostic methods like echocardiography. Many breast cancer patients are unable to undergo echocardiography due to surgery wounds and implants in their chest, making alternative diagnostic tools imperative[12].
Machine learning (ML) algorithms have shown remarkable progress in healthcare, including disease diagnosis, treatment, and prognosis. These advancements are particularly beneficial for developing predictive models that can assist in the early detection of cardiac disorders resulting from cancer treatments[8]. In this context, ML-driven analysis of CTG data can offer an alternative means of monitoring cardiac health tailored to meet the unique needs of breast cancer patients who might not otherwise have access to reliable cardiac assessment tools.
Furthermore, the development of AI and deep learning models that interpret CTG data has the potential to significantly improve the monitoring and management of patients undergoing chemotherapy. By accurately predicting cardiovascular issues, these technologies can facilitate timely interventions and ultimately enhance patient outcomes[2][10]. Given the growing focus on cardio-oncology, the integration of machine learning in this field underscores a significant shift towards more personalized and accessible healthcare solutions for breast cancer patients.
Application in Diabetes
The integration of advanced machine learning (ML) algorithms within healthcare has been pivotal in transforming how various diseases, including diabetes, are diagnosed and managed. Techniques employing ML are becoming essential for improving workflow and performance in clinical practice, enhancing the accuracy of early disease diagnosis, and developing patient-tailored therapies[3]. Specifically, ML algorithms have the potential to revolutionize diabetes management by providing more precise risk assessments and personalized treatment plans, leading to improved prognosis for patients[3].
The utilization of ML algorithms for diabetes care involves analyzing vast amounts of data to identify patterns and predict outcomes, which can inform treatment decisions. For instance, predictive models can assess an individual's risk of developing diabetes-related complications, thereby allowing healthcare providers to implement preventive strategies at an earlier stage[3]. Moreover, the genomic assessment of diabetes using ML techniques could lead to the identification of genetic markers associated with the disease, further advancing precision medicine[3].
Incorporating these algorithms into diabetes management systems can also facilitate continuous monitoring and real-time analysis of patient data, providing valuable insights into blood glucose trends and responses to different treatment regimens. This capability supports healthcare professionals in making informed decisions, ultimately enhancing patient outcomes and quality of life[3]. As such, the advancement of ML in diabetes care exemplifies the broader potential of these technologies to address complex healthcare challenges and improve patient care across various domains.
Results and Analysis
The study investigates the application of advanced machine learning algorithms in analyzing cardiotocography (CTG) data to assess fetal health, particularly in the contexts of breast cancer and diabetes. Various machine learning methods, including both deep learning and traditional approaches, were utilized to process and interpret the data, with performance metrics such as accuracy, specificity, sensitivity, F-measure, and ROC curve being evaluated using the WEKA software[10].
The cardiotocography data, sourced from the UCI database and processed through the SISPORTO 2.0 software, were analyzed by three expert obstetricians to classify the fetal condition as normal or pathological[10]. In the comparative study of machine learning models, a CNN model achieved an accuracy of 94.5%, while a Random Forests (RF) model attained a slightly higher accuracy of 96.2%[11]. This suggests that while the ensemble approach using RF might offer a marginal advantage in this specific context, individual models also demonstrate robust performance.
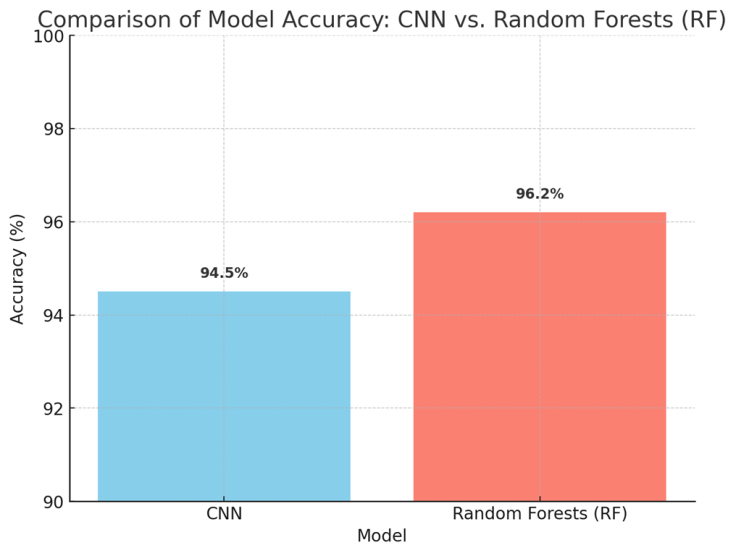
The study followed the PRISMA-ScR guidelines for systematic reviews and included a comprehensive search in databases such as Ovid Medline and PubMed, alongside manual searches of reference lists[2]. The search strategy employed terms related to fetal monitoring, machine learning, and associated fields to gather relevant literature[2]. This rigorous methodological approach ensures that the results are grounded in a broad spectrum of current research and practice.
Challenges and Limitations
The application of advanced machine learning algorithms to identify and explore fetal health based on cardiotocography (CTG) data presents several challenges and limitations. One significant challenge is the variability in the quality and consistency of CTG data interpretation. Although AI-assisted interpretation has the potential to reduce observer variability, this has not yet been demonstrated in randomized controlled trials, highlighting a gap in the evidence base that needs addressing[2].
Another limitation is the heterogeneity of data used in developing these algorithms. Studies conducted in high-income countries may not translate well to other settings due to differences in available resources and medical practices[4]. This poses a challenge in generalizing the findings to a broader context.
Additionally, the evidence supporting the impact of training on CTG interpretation for improving neonatal and maternal outcomes is limited and of low overall quality. There is a need for better-designed studies to establish the optimal content and method of delivering such training[13]. The inconsistent effects observed in the existing evidence indicate that current training methods may not be effective in all settings.
Moreover, the presence of maternal medical conditions, such as diabetes or hypertension, can complicate the use of CTG and the interpretation of its results[4][14]. These conditions introduce variability in fetal heart rate patterns, which may affect the accuracy of machine-learning algorithms. Thus, the development of algorithms that can account for such variables remains a critical need.
Lastly, the challenges extend to ethical and legal implications. The reduction of observer variability through AI has potential medical-legal consequences, particularly in reducing litigation related to fetal monitoring errors[2]. Therefore, robust validation and ethical considerations must be integrated into the deployment of these advanced algorithms in clinical practice.
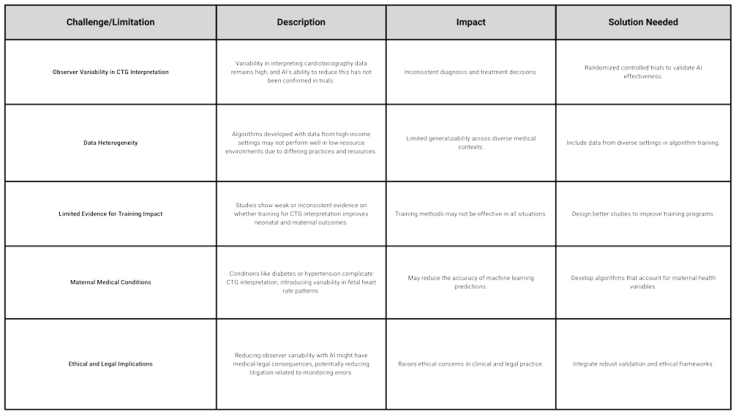
Future Research Directions
Future research in the field of fetal health monitoring through cardiotocography (CTG) and machine learning should focus on several key areas to address current limitations and enhance clinical utility. Firstly, there is a need for a comparative analysis of the accuracy of physiological measures used to detect and monitor fetal health across different populations, as existing studies have predominantly been conducted in high-income countries, which may not translate effectively to other healthcare settings[4]. This analysis could reveal disparities and inform the adaptation of algorithms to diverse demographic and clinical environments.
Additionally, there is a pressing requirement to bridge the gap in the literature regarding the computerized interpretation of CTG, particularly through the development of artificial intelligence (AI) and deep learning models[2]. Although advances in AI have improved diagnostic accuracy in various medical fields, the literature on its application to CTG remains sparse[2]. Enhancing AI algorithms could improve the reliability of distinguishing between healthy and pathological fetal states by integrating traditional and nonlinear fetal heart rate features into the models[5].
Furthermore, future research should aim to refine the predictive accuracy of these AI algorithms, especially in identifying the suspicious state of the fetus, which current models have struggled to achieve[9]. This could involve developing more sophisticated machine learning frameworks that incorporate a wider range of data points and diagnostic criteria to enhance their performance[3].
Research should also explore the potential of integrating CTG data with other physiological and diagnostic data, such as ultrasound, to create a more comprehensive fetal health assessment tool. This integration could help in developing a more holistic approach to fetal monitoring, ultimately improving maternal and fetal outcomes[11].
Finally, interdisciplinary collaboration between medical and engineering professionals should continue to be fostered to ensure that the development of these technologies aligns with clinical needs and practices. By focusing on these research directions, the medical community can leverage machine learning to its full potential, advancing the diagnosis and management of fetal health conditions, with implications extending to related fields such as breast cancer and diabetes management[9][15].
References
[1] Tidy, C., & McKechnie, D. (2024, October 10). Cardiotocography. Patient. https://patient.info/pregnancy/cardiotocography
[2] Aeberhard, J. L., Radan, A.-P., Delgado-Gonzalo, R., Strahm, K. M., Sigurthorsdottir, H. B., Schneider, S., & Surbek, D. (2022). Artificial intelligence and machine learning in cardiotocography: A scoping review. Journal of Obstetrics and Gynaecology. https://doi.org/10.1016/j.ejogrb.2022.12.005
[3] Cuocolo, R., Perillo, T., De Rosa, E., Ugga, L., & Petretta, M. (2019). Current applications of big data and machine learning in cardiology. Journal of Geriatric Cardiology, 16(8), 601–607. https://doi.org/10.11909/j.issn.1671-5411.2019.08.002
[4] Alfirevic, Z., Gyte, G. M. L., & Devane, D. (2015). Antenatal cardiotocography for fetal assessment. Cochrane Database of Systematic Reviews, 2015(9), CD007863. https://doi.org/10.1002/14651858.CD007863.pub4
[5] Ponsiglione, A. M., Cosentino, C., Cesarelli, G., Amato, F., & Romano, M. (2021). A Comprehensive Review of Techniques for Processing and Analyzing Fetal Heart Rate Signals. Sensors, 21(18), 6136. https://doi.org/10.3390/s21186136
[6] Heelan, L. (2013). Fetal Monitoring: Creating a Culture of Safety With Informed Choice. Journal of Perinatal Education, 22(3), 156–165. https://doi.org/10.1891/1058-1243.22.3.156
[7] Ayres-de-Campos, D. (2018). Electronic fetal monitoring or cardiotocography, 50 years later: What's in a name? American Journal of Obstetrics and Gynecology, 218(6), 545–546. https://doi.org/10.1016/j.ajog.2018.03.011
[8] Mehbodniya, A., Lazar, A. J. P., Webber, J., Sharma, D. K., Jayagopalan, S., Kittusamy, K., Singh, P., Rajan, R., Pandya, S., & Sengan, S. (2021). Fetal health classification from cardiotocographic data using machine learning. ResearchGate. https://www.researchgate.net/publication/356666279_Fetal_health_classification_from_cardiotocographic_data_using_machine_learning
[9] Hoodbhoy, Z., Noman, M., Shafique, A., Nasim, A., Chowdhury, D., & Hasan, B. (2019). Use of Machine Learning Algorithms for Prediction of Fetal Risk using Cardiotocographic Data. International Journal of Applied and Basic Medical Research, 9(4), 226–230. https://doi.org/10.4103/ijabmr.IJABMR_370_18
[10] Wu, Q., Wang, Y. & Lü, Z. (2015). A tabu search based hybrid evolutionary algorithm for the max-cut problem. Applied Soft Computing, 33, 231–238. https://doi.org/10.1016/j.asoc.2015.04.033
[11] Yalamanchili, S., Mohanty, S. N., Venkata, J., Chalapathi, M. M. V., & others. (2024). Cardiotocography Data Analysis for Fetal Health Classification Using Machine Learning Models. IEEE Access, PP(99). https://doi.org/10.1109/ACCESS.2024.3364755
[12] Harada, Y., Shimada, K., Harada, S. J., Sato, T., Kubota, Y., & Yamashita, M. (2024). Comparison of Echocardiography and Myocardial Scintigraphy to Detect Cancer Therapy-Related Cardiovascular Toxicity in Breast Cancer Patients. Journal of Imaging, 10(3), Article 54. https://doi.org/10.3390/jimaging10030054
[13] Kelly, S., Redmond, P., King, S., Oliver-Williams, C., Lamé, G., Liberati, E., Kuhn, I., Winter, C., Draycott, T., Dixon-Woods, M., & Burt, J. (2021). Training in the use of intrapartum electronic fetal monitoring with cardiotocography: systematic review and meta-analysis. BJOG: An International Journal of Obstetrics & Gynaecology, 128(9), 1408–1419. https://doi.org/10.1111/1471-0528.16619
[14] Grivell, R. M., Alfirevic, Z., Gyte, G. M. L., & Devane, D. (2015, September 12). Cardiotocography (a form of electronic fetal monitoring) for assessing a baby's well-being in the womb during pregnancy. Cochrane Database of Systematic Reviews. https://www.cochrane.org/CD007863/PREG_cardiotocography-form-electronic-fetal-monitoring-assessing-babys-well-being-womb-during-pregnancy
[15] McNally, R. J., Kotz, S. A., Wilhelm, F. H., & Hamlin, J. K. (n.d.). Physiological Measure. In Psychology topics. ScienceDirect. Retrieved October 14, 2024, https://www.sciencedirect.com/topics/psychology/physiological-measure