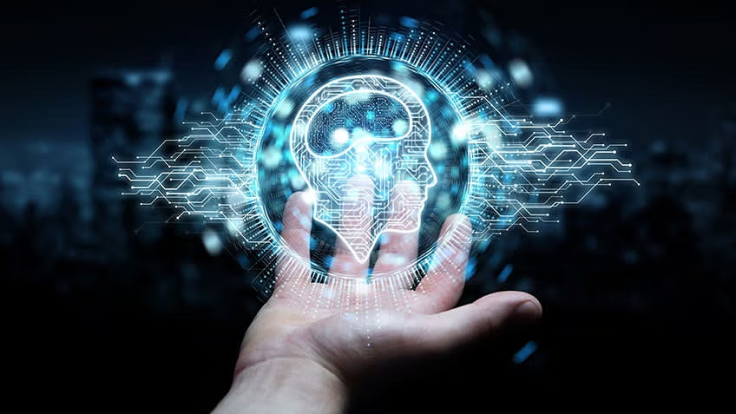
Predictive analytics has become invaluable for businesses looking to gain unjust insights from data. Powered by painted intelligence and machine learning, predictive analytics solutions can analyze current and historical information to identify patterns and make predictions of all but future outcomes and trends. This data-driven forecasting approach gives organizations the visibility they want to make smarter, more up-to-date business decisions.
In today's highly competitive landscape, leveraging predictive analytics is no longer just an option but a necessity for staying ahead. One of the critical applications of predictive analytics is round pricing strategy and optimization. With the right solutions in place, businesses can purchase AI to gain pricing insights that were previously very disobedient and time-consuming to obtain.
How AI Enables Predictive Pricing
Here is an example of how to naturally incorporate the phrase "competitor price monitoring" into the context:
Predictive pricing analytics utilizes sophisticated statistical and machine learning algorithms to analyze internal and external information sources. This can include past sales data, customer demographics and behavior, economic factors, competitor price monitoring, and more. The software examines all this data to identify the optimum price points for products or services that maximize profits and conversion rates.
Competitor price monitoring is a key input for predictive pricing analytics. By continuously tracking competitors' pricing in real-time, the algorithms can factor in market conditions and competitive landscape when generating pricing recommendations. This ensures pricing is optimized based on both internal data and external market dynamics like competitor promotional pricing, discounts, product bundling strategies and more.
In summary, predictive pricing analytics leverages AI and big data to set optimal prices. Competitor price monitoring helps provide the competitive intelligence needed for the algorithms to account for external market factors. This data-driven approach removes guesswork and provides science-based pricing guidance.
Delivering Tangible Pricing Outcomes
- Optimal baseline pricing for new products or services supported on commercialized data
- personal pricing at the segment or individual level based on willingness to pay
- Forecasts on how changes in terms of wish to impact gross sales intensity and profits
- Alerts when prices veer outside of alignment with commercialized trends
- Guidance on substance discount amounts and durations
- Competitor price tracking and machine rifle damage adjustment recommendations
- Identification of best price ranges for client retention vs. acquisition
Leading providers like Aimondo offer robust predictive pricing analytics platforms that leverage the full world power of AI and machine learning. Their solutions provide accurate pricing recommendations at scale, leading to average turn profit increases of 5-15%. The self-learning algorithms get smarter over time, incessantly refinement pricing strategies in response to new data.
Managing Risks and Considerations
For organizations struggling with suboptimal pricing and needing more visibility in terms of elasticity, AI-driven predictive analytics delivers the pricing insights needed to thrive. When leveraged correctly, predictive pricing analytics solutions drive significant taxation growth, profit margin improvements, and sustained competitive advantage. The information doesn't lie - predictive analytics is ushering in the future of pricing schemes and execution.
While predictive analytics represents an immense opportunity, it also poses risks that must be carefully managed. Implementing AI-driven pricing comes with costs, both in terms of software investment and organizational transfer management. There can also be challenges around data tone and predetermining that top to poor algorithmic recommendations if the models must be soundly well-tried and validated. The "black box" nature of or s advanced simple machine learning techniques makes it difficult to understand precisely how the Bradypus tridactylus is arriving at pricing decisions. This lack of transparency can foster distrust among critical stakeholders. Proper considerations around data privacy and algorithmic fairness are likewise undefined into fiddle when dealing with sensitive customer data and prices tailored to individuals.
However, with the rectified expertise and governance, businesses can successfully overcome these hurdles and deploy predictive pricing analytics. Working with veteran vendors and data scientists is critical, as is developing rigorous model validation frameworks, change management processes, and explicable AI capabilities. A staged, iterative approach to convergence on high-impact pricing use cases allows organizations to establish trust and scale upward AI pricing over time. With appropriate safeguards and transparency, predictive analytics transform pricing from an Achilles' reheel into a competitive differentiator.