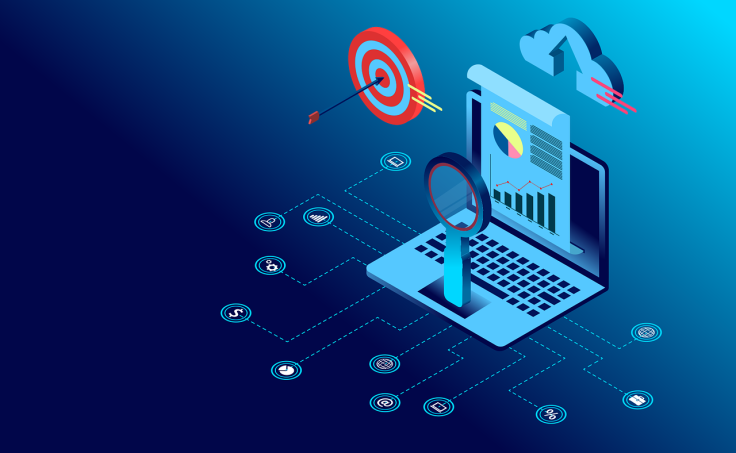
When businesses want to increase customer satisfaction, boost campaign success, and improve their bottom line, where do they look? Over the past few years, the answer has become increasingly clear: data. Data comes in many forms, including internal metrics, customer-facing interaction data, and big data. The latter of these refers to extremely voluminous sets of data that businesses can use to reveal widespread trends, patterns, or ideas in consumer markets or entire industries.
Big data provides critical insight into the wider context of a market, allowing businesses to respond more effectively to the change in the world and adapt their products and offerings. At present, around 97% of businesses have already invested in big data. The reason this figure is so astoundingly high is that this insight isn't a luxury but rather a necessity.
Yet, as more businesses attempt to contend with growing datasets, it's becoming difficult to manage big data workloads. When processing potentially millions of data points on a daily basis to deduce critical insight and trends, businesses have to push their resources to the max to be able to manage.
In this article, we'll dive into the world of big data management, comment on the core challenges that businesses experience, and outline exactly how to overcome each of them.
What Are the Core Challenges and Solutions in Big Data Workload Management
The main problem that any business will face when interacting and using big data is exactly that—its size. Big data isn't a dataset that one person can quickly read through and draw insight from. On the contrary, it is comprised of datasets that are far too large for any traditional data processing software to deal with. Instead of a dataset that refers to a company's performance, big data would look at an entire country's performance represented through every business in that area.
To effectively process and manage big data, businesses must enlist platforms, tools, and data architecture that offer the highest level of statistical analysis and processing power. With this task comes a range of potential problems. Let's explore each of these in turn and suggest methods that your business can use to overcome them.
Managing Growing Volumes of Data
Beyond just the size of big data sets, businesses must also be able to manage the fact that they are continuously expanding. Big data is constantly gathering more information and processing further insight to give additional context to its datasets. In order to manage this growing volume of data, businesses need to have the capacity to scale their management facilities as quickly as possible.
Data volume is a key concern when engaging with big data management. Beyond just collecting this data, businesses must also be able to store it and manage the growing volume. Instead of segmenting your data into various silos that could reduce the overall cohesion of your datasets, your business should instead look to modern data architecture that can effectively scale with your company.
One effective example of this is looking into any cloud data warehouse solutions that offer scalable plans for data storage. The vast majority of suppliers will allow your business to simply expand the amount of data that it interacts with and stores, helping to manage the growing volume without much hassle.
Another potential solution that your business could opt for is to use a distributed storage system that makes use of horizontal scaling to manage more data. By distributing data across several nodes, your business can continuously increase the total amount of information it manages through strategies like partitioning and lifetime scaling.
Real-Time Data Processing
One area of analytics that is becoming increasingly utilized in the world of business operations is real-time data processing and live analytics. Traditional forms of data processing will use batch processing to ensure the timely delivery of insight. This approach isn't functional with big data analytics due to the sheer volume of data that businesses must move through the data pipeline at any one given moment.
Instead of using legacy strategies, businesses should instead focus on using stream processing frameworks to handle these larger data streams. These frameworks typically directly pair with leading cloud storage offerings by leading providers, allowing your business to process data more effectively as it becomes available to your business.
Another potential solution is to rely on in-memory databases, as these will be able to process data more rapidly, delivering the insight you need in a shorter span of time.
Maintaining Data Quality
Whatever scale of data your business is working with, data quality is going to be a central issue that you must contend with. This is especially true when working with big data, as you have greater volumes of data to check and ensure it is up to standard. Data quality is a general term that refers to the overall accuracy of data.
Another important aspect of data quality to consider when dealing with big data is its relevance to your business. When dealing with mammoth datasets, you're more likely to encounter data that simply does not apply to your business objectives and would only clutter your data pipeline with pointless information.
When attempting to improve data quality when dealing with big data management, your business should move toward automation strategies. Part of the impossibility of dealing with big data is the fact that it's too big for people to engage with on an individual level. With that in mind, automation is a useful strategy as you can implement data quality checks along your data pipeline.
When new sources of big data enter your business, your automated strategies will help to ensure its quality before it ever becomes a resource, cost, or time issue for your company.
Final Thoughts
Big data isn't going anywhere. On the contrary, its usage and critical importance to a range of industries will only become more pronounced over the next few years. Instead of attempting to use the same data architecture to contend with the growing wave of big data, businesses must be able to adapt to the challenges they face on the road.
By exploring and understanding the key challenges that a company is likely to face when managing big data workloads, it is much more likely to be able to overcome these roadblocks. Following the advice in this article, a business will be able to improve its existing data infrastructure and overcome the common challenges it will face along the way.
Best of luck honing your big data strategies in 2024!