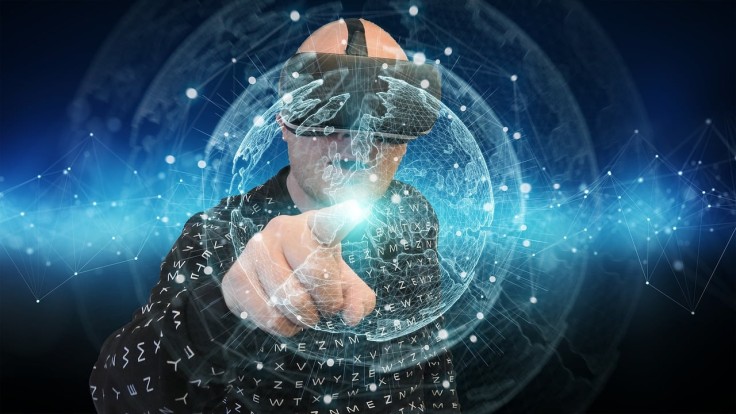
With the rapid growth of AI and Natural Language Processing, the merging of Retrieval-Augmented Generation (RAG) has become a major breakthrough in this field. RAG's language generation models integrate the security of traditional models along with access to overwhelming data sources, offering a more connected, competent, and relevant generation of outputs. The combination of these retrieval and generation factors has, in turn, revealed novel avenues for developing imaginative ideas and solving problems that correspond to the competing fields of content creation and scientific research.
In the following article, we venture into the fascinating realm of enhanced retrieval innovations and explore their uses and advantages that we may realize as we look to the future.
The Evolution of Natural Language Processing
Natural language processing (NLP) has witnessed a drastic development trend these years, keeping up with the progress in machine learning algorithms, availability of big data, and so on. Modern language models like GPT series from OpenAI have been fine examples of how great artificial neural networks can be in generating human-like text when given input prompt. However, these models compose their answers relying solely and simply on their own built-in knowledge without any interaction with people.
Retrieval-Augmented Generation: A Paradigm Shift
Retrieval-augmented generation's advent revolutionizes and reorients NLP research in a breakthrough manner. RAG systems, unlike traditional models that rely solely on memory, can access diverse sources such as structured databases or unstructured text corpora. This capability enables them to retrieve information from these sources and integrate relevant facts into the generation process; consequently enhancing output quality and relevance.
Similar to RAG, the first AI implementation that is worth mentioning is OpenAI's Codex. Trained on diverse data sources including code repositories and program forums, Codex offers superior proficiency over other models when it comes to accessing an extensive databank of programming information for precise translations. Integrating retrieval mechanisms enables Codex to retrieve pertinent code patterns and adapt them for specific tasks. This accelerates the software development process and empowers developers with instant assistance.
Applications Across Domains
The applications of retrieval-augmented generation span diverse domains, showcasing its versatility and potential impact. In content creation, RAG tool can assist writers, journalists, and marketers by providing relevant information, generating outlines, and suggesting creative ideas. For example, a content creator working on an article about renewable energy could use an RAG model to retrieve up-to-date statistics, relevant research papers, and compelling arguments, facilitating the writing process and ensuring accuracy.
Reference Management (RAG) systems hold immense promise in accelerating and enhancing scientific discovery. Indeed, researchers may not only harness RAG systems to shorten the time frame of their investigations but also improve their effectiveness. These innovative tools enable them to dive into vast collections of academic papers, retrieving relevant resources for hypothesis formulation based on existing knowledge. Consequently, this methodology goes beyond merely curtailing literature redundancy and repetition; it consolidates collaborative ventures while facilitating new idea formulation—thereby yielding a more robust outcome.
Ethical Considerations and Challenges
Despite its potential benefits, retrieval-augmented generation also raises ethical considerations and challenges. Incorporating different knowledge sources that are present outside creates the issue of data bias and misinformation as well as privacy concerns. Developers need to select and verify the sets on which their RAG models will be trained closely with the data librarians. They must ensure the representativeness of datasets and pay attention to the fact that they adhere to ethical standards.
Furthermore, the spread of RAG technology brings into question the issues of intellectual property rights and content ownership. Generative AI is on approach to the point where information can be easily sourced and remixed from a wide variety of different sources, raising the issue of how attribution shall be given and whether it would even be a case of plagiarism or fair use. Notions of clear standards and policies must be developed to deal with these issues and to foster a responsible culture among AI experts.
The Future of Creativity and Innovation
Glancing into the future, the advancement of the augmented generation retrieval process is likely to be very beneficial in determining the future of creativity and innovation. As RAG systems keep evolving, they assist people and entities in freeing their creative resources, overcoming cognitive obstacles, and addressing sophisticated problems at record speeds. RAG, through the pooling of humanity's collective intelligence, opens up new prospects in content creation, research, education, and more generally.
As the last thought, retrieval-augmented generation, being a breakthrough in natural language processing, is a turning point in the gap between AI and human intelligence. Through the combination of generation and retrieval components, RAG systems are able to open new horizons of creativity, collaboration, and discovery. Through the course of this journey, we are embarking on the uncharted terrain of AI technologies; the benefits of RAG may be the thing that changes the way we deal with knowledge.